artificial intelligence
This New Technique Slashes AI Energy Use by 95%
Published
1 month agoon
By
admin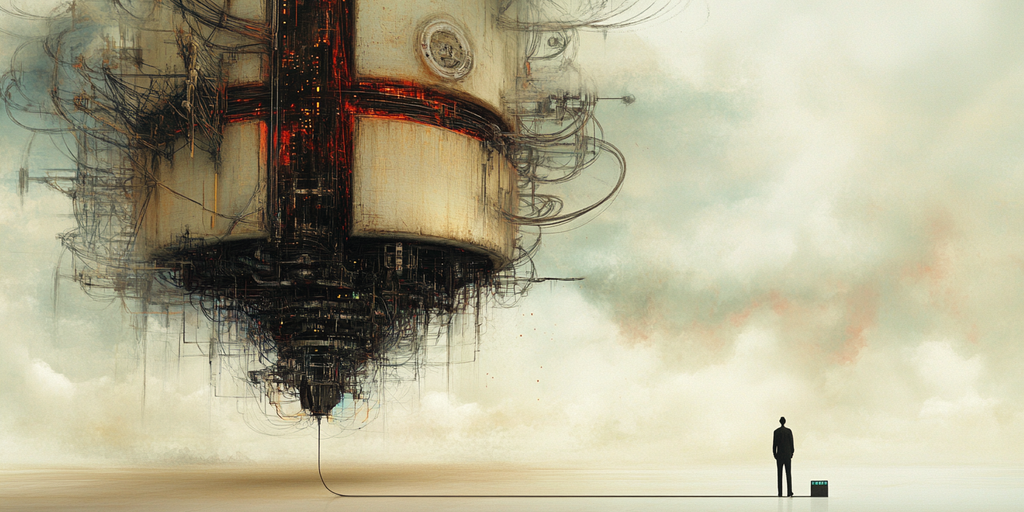
A new technique could put AI models on a strict energy diet, potentially cutting power consumption by up to 95% without compromising quality.
Researchers at BitEnergy AI, Inc. have developed Linear-Complexity Multiplication (L-Mul), a method that replaces energy-intensive floating-point multiplications with simpler integer additions in AI computations.
For those unfamiliar with the term, floating-point is a mathematical shorthand that allows computers to handle very large and very small numbers efficiently by adjusting the placement of the decimal point. You can think of it like scientific notation, in binary. They are essential for many calculations in AI models, but they require a lot of energy and computing power. The bigger the number, the better the model is—and the more computing power it requires. Fp32 is generally a full precision model, with developers reducing precision to fp16, fp8, and even fp4, so their models can run on local hardware.

AI’s voracious appetite for electricity has become a growing concern. ChatGPT alone gobbles up 564 MWh daily—enough to power 18,000 American homes. The overall AI industry is expected to consume 85-134 TWh annually by 2027, roughly the same as Bitcoin mining operations, according to estimations shared by the Cambridge Centre for Alternative Finance.
L-Mul tackles the AI energy problem head-on by reimagining how AI models handle calculations. Instead of complex floating-point multiplications, L-Mul approximates these operations using integer additions. So, for example, instead of multiplying 123.45 by 67.89, L-Mul breaks it down into smaller, easier steps using addition. This makes the calculations faster and uses less energy, while still maintaining accuracy.
The results seem promising. “Applying the L-Mul operation in tensor processing hardware can potentially reduce 95% energy cost by element wise floating point tensor multiplications and 80% energy cost of dot products,” the researchers claim. Without getting overly complicated, what that means is simply this: If a model used this technique, it would require 95% less energy to think, and 80% less energy to come up with new ideas, according to this research.
The algorithm’s impact extends beyond energy savings. L-Mul outperforms current 8-bit standards in some cases, achieving higher precision while using significantly less bit-level computation. Tests across natural language processing, vision tasks, and symbolic reasoning showed an average performance drop of just 0.07%—a negligible tradeoff for the potential energy savings.
Transformer-based models, the backbone of large language models like GPT, could benefit greatly from L-Mul. The algorithm seamlessly integrates into the attention mechanism, a computationally intensive part of these models. Tests on popular models such as Llama, Mistral, and Gemma even revealed some accuracy gain on certain vision tasks.
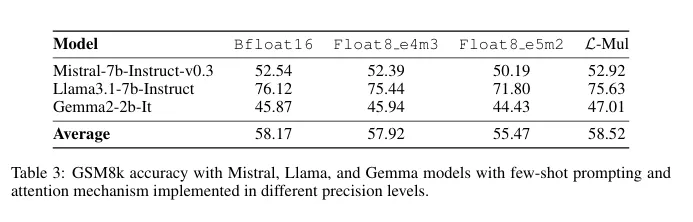
At an operational level, L-Mul’s advantages become even clearer. The research shows that multiplying two float8 numbers (the way AI models would operate today) requires 325 operations, while L-Mul uses only 157—less than half. “To summarize the error and complexity analysis, L-Mul is both more efficient and more accurate than fp8 multiplication,” the study concludes.
But nothing is perfect and this technique has a major achilles heel: It requires a special type of hardware, so the current hardware isn’t optimized to take full advantage of it.
Plans for specialized hardware that natively supports L-Mul calculations may be already in motion. “To unlock the full potential of our proposed method, we will implement the L-Mul and L-Matmul kernel algorithms on hardware level and develop programming APIs for high-level model design,” the researchers say. This could potentially lead to a new generation of AI models that are fast, accurate, and super cheap—making energy-efficient AI a real possibility.
Generally Intelligent Newsletter
A weekly AI journey narrated by Gen, a generative AI model.
Source link
You may like
How Viable Are BitVM Based Pegs?
UK Government to Draft a Regulatory Framework for Crypto, Stablecoins, Staking in Early 2025
Bitcoin Cash eyes 18% rally
Rare Shiba Inu Price Patterns Hint SHIB Could Double Soon
The Bitcoin Pi Cycle Top Indicator: How to Accurately Time Market Cycle Peaks
Bitcoin Approaches $100K; Retail Investors Stay Steady
AI
Decentralized AI Project Morpheus Goes Live on Mainnet
Published
3 days agoon
November 19, 2024By
admin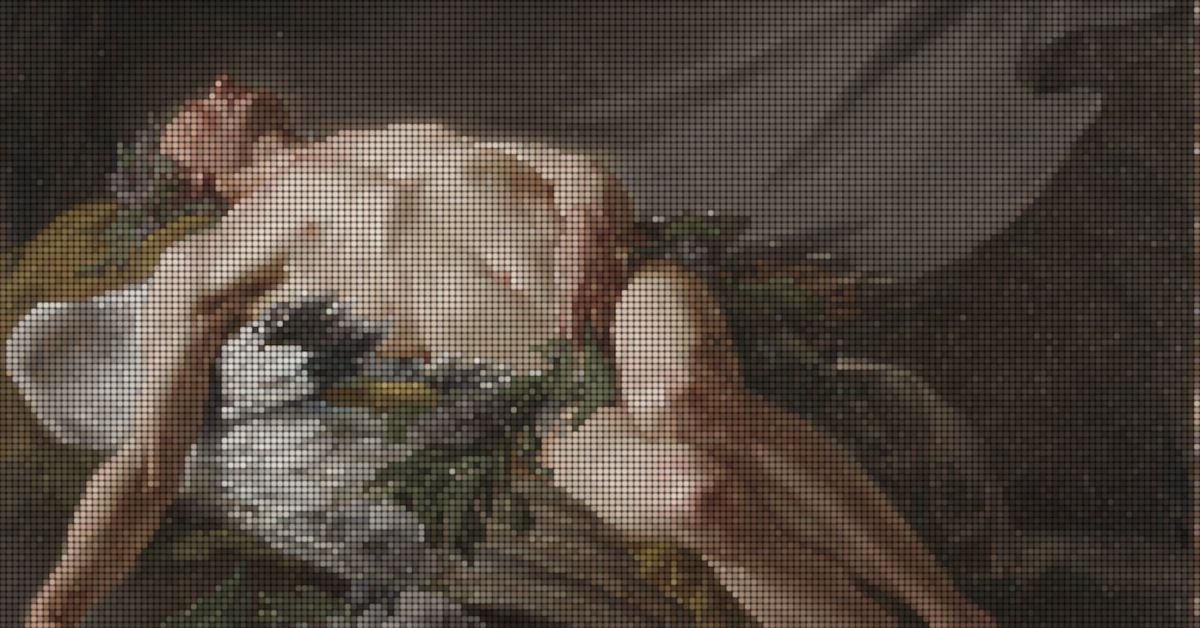

Morpheus went live on a public testnet, or simulated experimental environment, in July. The project promises personal AIs, also known as “smart agents,” that can empower individuals much like personal computers and search engines did in decades past. Among other tasks, agents can “execute smart contracts, connecting to users’ Web3 wallets, DApps, and smart contracts,” the team said.
Source link
artificial intelligence
How the US Military Says Its Billion Dollar AI Gamble Will Pay Off
Published
6 days agoon
November 16, 2024By
admin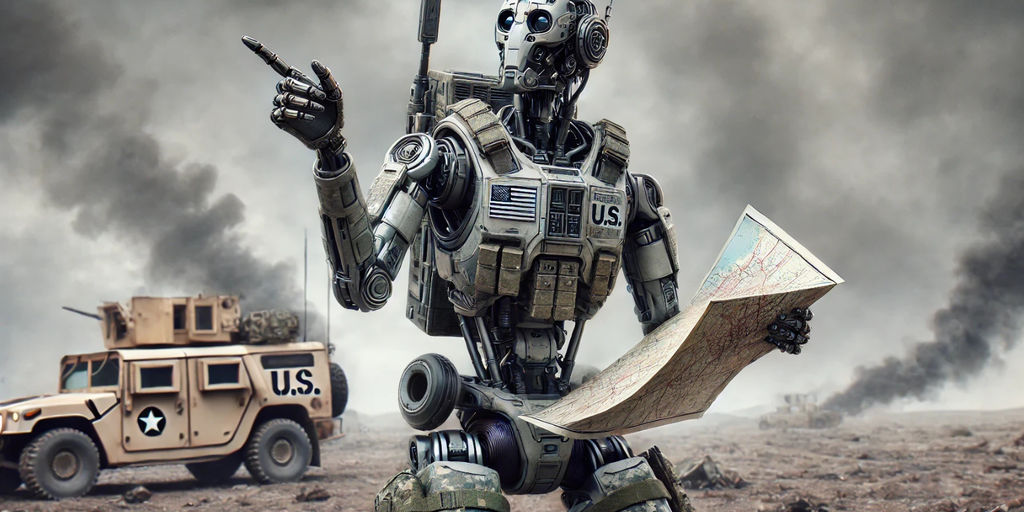
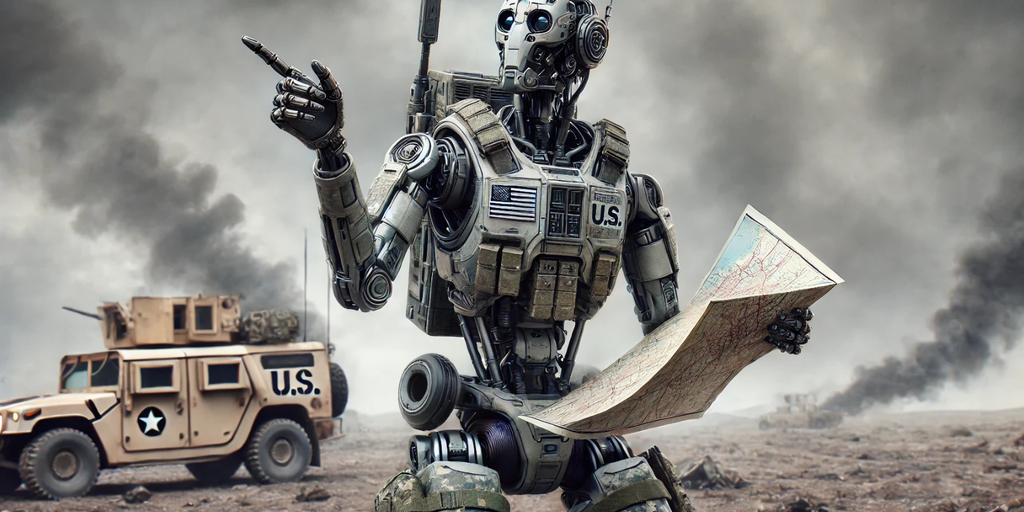
War is more profitable than peace, and AI developers are eager to capitalize by offering the U.S. Department of Defense various generative AI tools for the battlefields of the future.
The latest evidence of this trend came last week when Claude AI developer Anthropic announced that it was partnering with military contractor Palantir and Amazon Web Services (AWS) to provide U.S. intelligence and the Pentagon access to Claude 3 and 3.5.
Anthropic said Claude will give U.S. defense and intelligence agencies powerful tools for rapid data processing and analysis, allowing the military to perform faster operations.
Experts say these partnerships allow the Department of Defense to quickly adopt advanced AI technologies without needing to develop them internally.
“As with many other technologies, the commercial marketplace always moves faster and integrates more rapidly than the government can,” retired U.S. Navy Rear Admiral Chris Becker told Decrypt in an interview. “If you look at how SpaceX went from an idea to implementing a launch and recovery of a booster at sea, the government might still be considering initial design reviews in that same period.”
Becker, a former Commander of the Naval Information Warfare Systems Command, noted that integrating advanced technology initially designed for government and military purposes into public use is nothing new.
“The internet began as a defense research initiative before becoming available to the public, where it’s now a basic expectation,” Becker said.
Anthropic is only the latest AI developer to offer its technology to the U.S. government.
Following the Biden Administration’s memorandum in October on advancing U.S. leadership in AI, ChatGPT developer OpenAI expressed support for U.S. and allied efforts to develop AI aligned with “democratic values.” More recently, Meta also announced it would make its open-source Llama AI available to the Department of Defense and other U.S. agencies to support national security.
During Axios’ Future of Defense event in July, retired Army General Mark Milley noted advances in artificial intelligence and robotics will likely make AI-powered robots a larger part of future military operations.
“Ten to fifteen years from now, my guess is a third, maybe 25% to a third of the U.S. military will be robotic,” Milley said.
In anticipation of AI’s pivotal role in future conflicts, the DoD’s 2025 budget requests $143.2 billion for Research, Development, Test, and Evaluation, including $1.8 billion specifically allocated to AI and machine learning projects.
Protecting the U.S. and its allies is a priority. Still, Dr. Benjamin Harvey, CEO of AI Squared, noted that government partnerships also provide AI companies with stable revenue, early problem-solving, and a role in shaping future regulations.
“AI developers want to leverage federal government use cases as learning opportunities to understand real-world challenges unique to this sector,” Harvey told Decrypt. “This experience gives them an edge in anticipating issues that might emerge in the private sector over the next five to 10 years.
He continued: “It also positions them to proactively shape governance, compliance policies, and procedures, helping them stay ahead of the curve in policy development and regulatory alignment.”
Harvey, who previously served as chief of operations data science for the U.S. National Security Agency, also said another reason developers look to make deals with government entities is to establish themselves as essential to the government’s growing AI needs.
With billions of dollars earmarked for AI and machine learning, the Pentagon is investing heavily in advancing America’s military capabilities, aiming to use the rapid development of AI technologies to its advantage.
While the public may envision AI’s role in the military as involving autonomous, weaponized robots advancing across futuristic battlefields, experts say that the reality is far less dramatic and more focused on data.
“In the military context, we’re mostly seeing highly advanced autonomy and elements of classical machine learning, where machines aid in decision-making, but this does not typically involve decisions to release weapons,” Kratos Defense President of Unmanned Systems Division, Steve Finley, told Decrypt. “AI substantially accelerates data collection and analysis to form decisions and conclusions.”
Founded in 1994, San Diego-based Kratos Defense has partnered extensively with the U.S. military, particularly the Air Force and Marines, to develop advanced unmanned systems like the Valkyrie fighter jet. According to Finley, keeping humans in the decision-making loop is critical to preventing the feared “Terminator” scenario from taking place.
“If a weapon is involved or a maneuver risks human life, a human decision-maker is always in the loop,” Finley said. “There’s always a safeguard—a ‘stop’ or ‘hold’—for any weapon release or critical maneuver.”
Despite how far generative AI has come since the launch of ChatGPT, experts, including author and scientist Gary Marcus, say current limitations of AI models put the real effectiveness of the technology in doubt.
“Businesses have found that large language models are not particularly reliable,” Marcus told Decrypt. “They hallucinate, make boneheaded mistakes, and that limits their real applicability. You would not want something that hallucinates to be plotting your military strategy.”
Known for critiquing overhyped AI claims, Marcus is a cognitive scientist, AI researcher, and author of six books on artificial intelligence. In regards to the dreaded “Terminator” scenario, and echoing Kratos Defense’s executive, Marcus also emphasized that fully autonomous robots powered by AI would be a mistake.
“It would be stupid to hook them up for warfare without humans in the loop, especially considering their current clear lack of reliability,” Marcus said. “It concerns me that many people have been seduced by these kinds of AI systems and not come to grips with the reality of their reliability.”
As Marcus explained, many in the AI field hold the belief that simply feeding AI systems more data and computational power would continually enhance their capabilities—a notion he described as a “fantasy.”
“In the last weeks, there have been rumors from multiple companies that the so-called scaling laws have run out, and there’s a period of diminishing returns,” Marcus added. “So I don’t think the military should realistically expect that all these problems are going to be solved. These systems probably aren’t going to be reliable, and you don’t want to be using unreliable systems in war.”
Edited by Josh Quittner and Sebastian Sinclair
Generally Intelligent Newsletter
A weekly AI journey narrated by Gen, a generative AI model.
Source link
artificial intelligence
AI Startup Hugging Face is Building Small LMs for ‘Next Stage Robotics’
Published
1 week agoon
November 12, 2024By
admin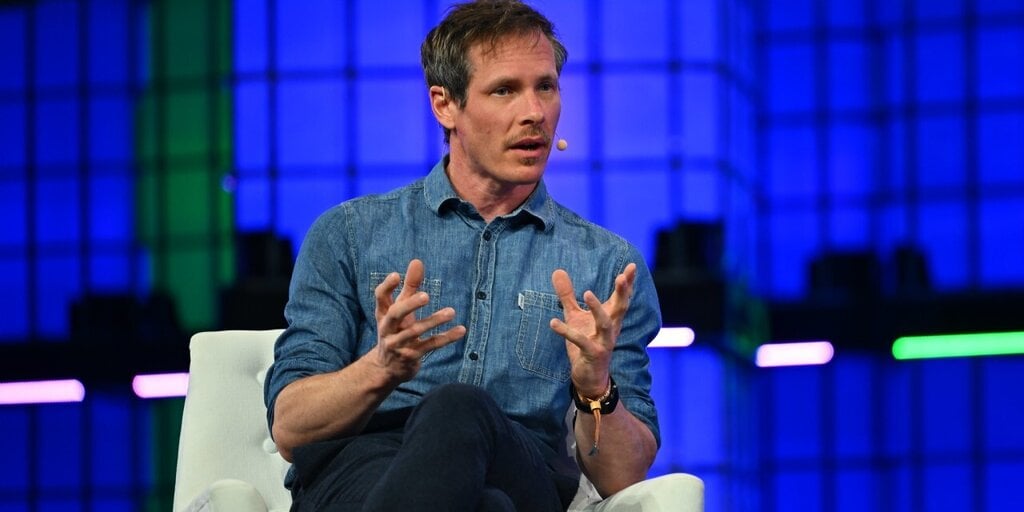
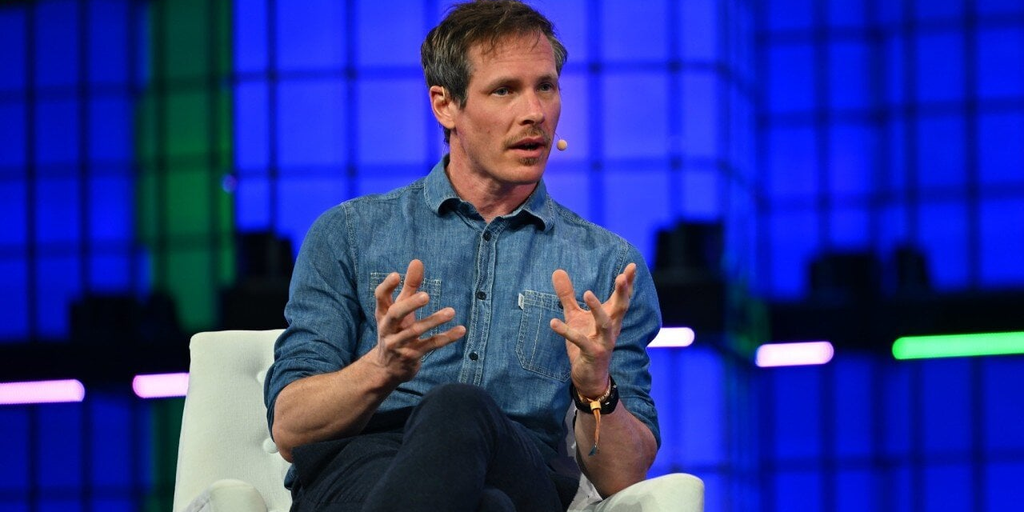
AI startup Hugging Face envisions that small—not large—language models will be used for applications including “next stage robotics,” its Co-Founder and Chief Science Officer Thomas Wolf said.
“We want to deploy models in robots that are smarter, so we can start having robots that are not only on assembly lines, but also in the wild,” Wolf said while speaking at Web Summit in Lisbon today. But that goal, he said, requires low latency. “You cannot wait two seconds so that your robots understand what’s happening, and the only way we can do that is through a small language model,” Wolf added.
Small language models “can do a lot of the tasks we thought only large models could do,” Wolf said, adding that they can also be deployed on-device. “If you think about this kind of game changer, you can have them running on your laptop,” he said. “You can have them running even on your smartphone in the future.”
Ultimately, he envisions small language models running “in almost every tool or appliance that we have, just like today, our fridge is connected to the internet.”
The firm released its SmolLM language model earlier this year. “We are not the only one,” said Wolf, adding that, “Almost every open source company has been releasing smaller and smaller models this year.”
He explained that, “For a lot of very interesting tasks that we need that we could automate with AI, we don’t need to have a model that can solve the Riemann conjecture or general relativity.” Instead, simple tasks such as data wrangling, image processing and speech can be performed using small language models, with corresponding benefits in speed.
The performance of Hugging Face’s LLaMA 1b model to 1 billion parameters this year is “equivalent, if not better than, the performance of a 10 billion parameters model of last year,” he said. “So you have a 10 times smaller model that can reach roughly similar performance.”
“A lot of the knowledge we discovered for our large language model can actually be translated to smaller models,” Wolf said. He explained that the firm trains them on “very specific data sets” that are “slightly simpler, with some form of adaptation that’s tailored for this model.”
Those adaptations include “very tiny, tiny neural nets that you put inside the small model,” he said. “And you have an even smaller model that you add into it and that specializes,” a process he likened to “putting a hat for a specific task that you’re gonna do. I put my cooking hat on, and I’m a cook.”
In the future, Wolf said, the AI space will split across two main trends.
“On the one hand, we’ll have this huge frontier model that will keep getting bigger, because the ultimate goal is to do things that human cannot do, like new scientific discoveries,” using LLMs, he said. The long tail of AI applications will see the technology “embedded a bit everywhere, like we have today with the internet.”
Edited by Stacy Elliott.
Generally Intelligent Newsletter
A weekly AI journey narrated by Gen, a generative AI model.
Source link

How Viable Are BitVM Based Pegs?

UK Government to Draft a Regulatory Framework for Crypto, Stablecoins, Staking in Early 2025

Bitcoin Cash eyes 18% rally

Rare Shiba Inu Price Patterns Hint SHIB Could Double Soon

The Bitcoin Pi Cycle Top Indicator: How to Accurately Time Market Cycle Peaks
Bitcoin Breakout At $93,257 Barrier Fuels Bullish Optimism

Bitcoin Approaches $100K; Retail Investors Stay Steady

Solana Hits New ATH On Huge Whale Accumulation, More Gains Ahead?

Microsoft Should Buy $78 Billion Worth of Bitcoin

Ethereum Believers May Be Staring Down Opportunity As ETH Reaches Another Low Against Bitcoin: CryptoQuant CEO

UK government is ready for crypto regulations next year

“Crypto Dad” Chris Giancarlo Emerges Top For White House Crypto Czar Role

Bitcoin Nears $100,000 As Trump Council Expected To Implement BTC Reserve

Know Your Missiles: Russia’s Experimental Hypersonic Missile Is A New Kind of Killing Machine

Polkadot investor predicts a 30,000% rally for this $0.04 token by 2025

182267361726451435

Top Crypto News Headlines of The Week

Why Did Trump Change His Mind on Bitcoin?

New U.S. president must bring clarity to crypto regulation, analyst says

Ethereum, Solana touch key levels as Bitcoin spikes

Bitcoin Open-Source Development Takes The Stage In Nashville

Will XRP Price Defend $0.5 Support If SEC Decides to Appeal?

Bitcoin 20% Surge In 3 Weeks Teases Record-Breaking Potential

Ethereum Crash A Buying Opportunity? This Whale Thinks So

Shiba Inu Price Slips 4% as 3500% Burn Rate Surge Fails to Halt Correction

‘Hamster Kombat’ Airdrop Delayed as Pre-Market Trading for Telegram Game Expands

Washington financial watchdog warns of scam involving fake crypto ‘professors’

Citigroup Executive Steps Down To Explore Crypto

Mostbet Güvenilir Mi – Casino Bonus 2024

Bitcoin flashes indicator that often precedes higher prices: CryptoQuant
Trending
- 2 months ago
182267361726451435
- 24/7 Cryptocurrency News3 months ago
Top Crypto News Headlines of The Week
- Donald Trump4 months ago
Why Did Trump Change His Mind on Bitcoin?
- News3 months ago
New U.S. president must bring clarity to crypto regulation, analyst says
- Bitcoin4 months ago
Ethereum, Solana touch key levels as Bitcoin spikes
- Opinion4 months ago
Bitcoin Open-Source Development Takes The Stage In Nashville
- Price analysis3 months ago
Will XRP Price Defend $0.5 Support If SEC Decides to Appeal?
- Bitcoin4 months ago
Bitcoin 20% Surge In 3 Weeks Teases Record-Breaking Potential